With DISGENET, you can easily unlock new insights and greatly accelerate your drug development journey.
Every year, more than 1 million papers enter PubMed in the biomedical field [1]. While medical knowledge doubles every 73 days and is increasing at an exponential rate with no evidence of slowing [2], drug discovery and development remain a time-consuming and costly process, characterized by high failure rates and great uncertainty [3]. One of the biggest challenges facing drug discovery companies is that 80% of biomedical data is currently unstructured within text documents [4]. This data flood has become unmanageable for human readers and has set the stage for AI tools such as Natural Language Processing (NLP) to assist with improving drug discovery and drug development.
What is NLP?
NLP is an AI technique to process and analyze texts automatically. NLP enables the transition from time-consuming, manual, and isolated curation of natural language data to automated, large-scale, standardized processes for text analysis [4]. In essence, NLP reads an extensive amount of documents for the users. It identifies and extracts relevant facts and relationships in a structured format suitable for fast review and analysis. NLP connects facts to synthesize knowledge and generate actionable insights.
How does DISGENET integrate NLP?
DISGENET is a platform populated with top-quality data generated with our state-of-the-art NLP technologies (F-score 92%) especially tuned to extract fine-grained information from biomedical texts. Our NLP tool relies on community-driven ontologies and terminologies that we carefully curated to standardize highly relevant data for drug discovery. It combines language models to detect mentions of diseases, genes, variants and chemicals and their semantic relationships. The high standardization of our data allows for seamless integration with other datasets, ensuring complete interoperability with your internal data.
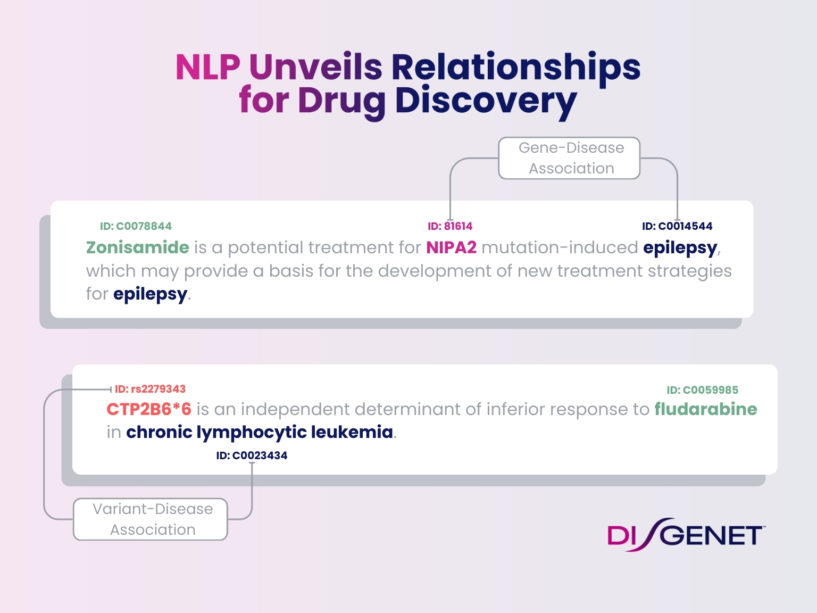
DISGENET leverages NLP to continually expand its pool of high-quality data with each quarterly update, creating a proprietary dataset spanning human and animal models (i.e., rat, mouse, fly, dog, and zebrafish among others). As a result, DISGENET structures up to date information with an ample coverage of diseases, phenotypes, genes, and variants which makes our platform an essential tool for drug development. DISGENET currently contains a corpus of over 1.5 million publications supporting genotype-phenotype associations, derived from analyzing over 30 million publications with NLP. Accessing DISGENET is equivalent to reading millions of articles to fully unlock the data.
For example, curated data sources report only 10 genes associated to Duchenne muscular dystrophy, while NLP data from DISGENET provides evidence for an additional 502 genes.
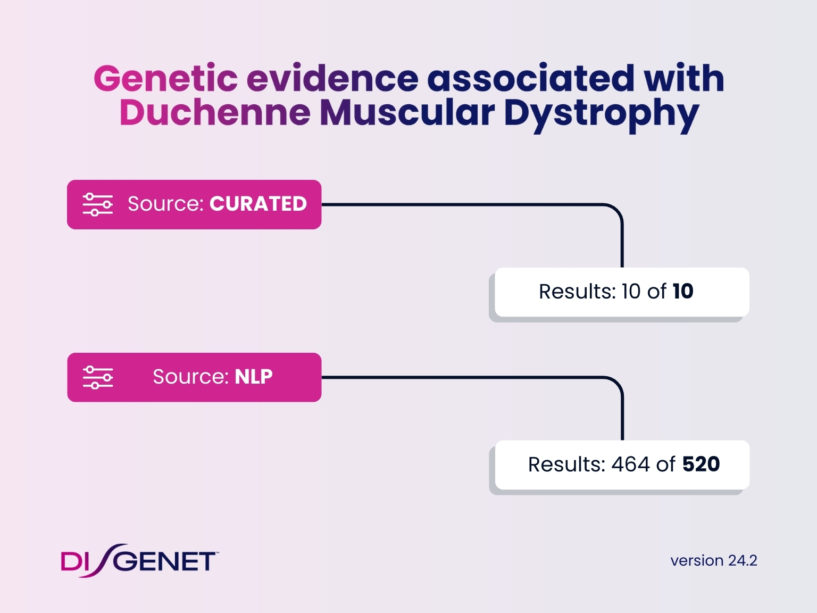
DISGENET v24.2 gathers over 50 million associations connecting:
- 700,000+ disease variants
- 40,000+ diseases & traits
- 32,000+ disease genes
How can DISGENET help you with your drug development research?
DISGENET and target identification in drug discovery
Ninety percent of drugs entering phase 1 clinical trials never reach the market due to efficacy or safety issues uncovered later on. These clinical failures highlight the great potential for improvement in selecting relevant targets. Drugs that modulate targets with supporting GWAS evidence are twice as likely to be approved [5]. Additionally, systematic analysis of FDA drug approvals showed that drugs with human genetics support were 2-5 fold more likely to lead to an improved therapy [6]. As a result, genetic evidence linking potential therapeutic targets and diseases is crucial for identifying and prioritizing targets leading to safe and effective drugs.
With DISGENET, you can:
- access to the genetic evidence that associates targets and diseases
- identify the targets with genetic evidence and their association types with a specific disease
- retrieve evidence for these targets from diverse sources and animal models
- identify rare genetic variations of the targets
- identify new targets by broadening the disease search using our proprietary ontology
DISGENET and safety assessment in drug development
Safety issues are a primary reason for drug candidate failure. However, genetic evidence can provide insights into target safety liabilities upfront. While genetic evidence supports many adverse events, the absence of negative consequences of gene loss of function unveils valuable insights into drug tolerability [7].
With DISGENET, you can:
- identify genetic variants of targets associated with adverse drug events or unwanted phenotypes
- identify the possible risks in modulating a specific target
- identify unwanted phenotypes associated with a target in various animal models
Read more about anticipating drug toxicity using DISGENET and chemical risk assessment with DISGENET
DISGENET and drug repurposing
Drug development is filled with uncertainty, marked by prolonged timelines to market and exorbitant costs. In that landscape, drug repurposing emerges as a great alternative to avoid the challenges associated with novel compounds development. Approved drugs have already passed all phases of clinical trials and have an established safety profile. Thus, their repurposing represents a major opportunity to rapidly access the market with reduced R&D risks, time, and costs [8].
With DISGENET, you can:
- identify diseases’ similarity based on their associated genes or semantic similarity
- identify new indications for the targets of an existing drug
DISGENET: proprietary scores and beyond for accelerated drug development
DISGENET provides highly relevant scores for drug discovery, allowing for easy navigation and prioritization of the data. We developed two scores to highlight the strength of gene-disease (GDA score), and variant-disease associations (VDA score). These scores range from 0 to 1 according to their level of evidence. They rely on the level of curation, the model organisms, and the number of publications reporting the association. Additionally, associations can be further characterized using association types. Our association type ontology includes drug response variants, causal mutation, or biomarkers among many others unveiling critical information for drug development.
Our NLP tool automatically highlights the key concepts in the relevant sentences of published abstracts. Gene, variant, disease, and chemical concepts, as well as any negation markers, are clearly displayed for your review.
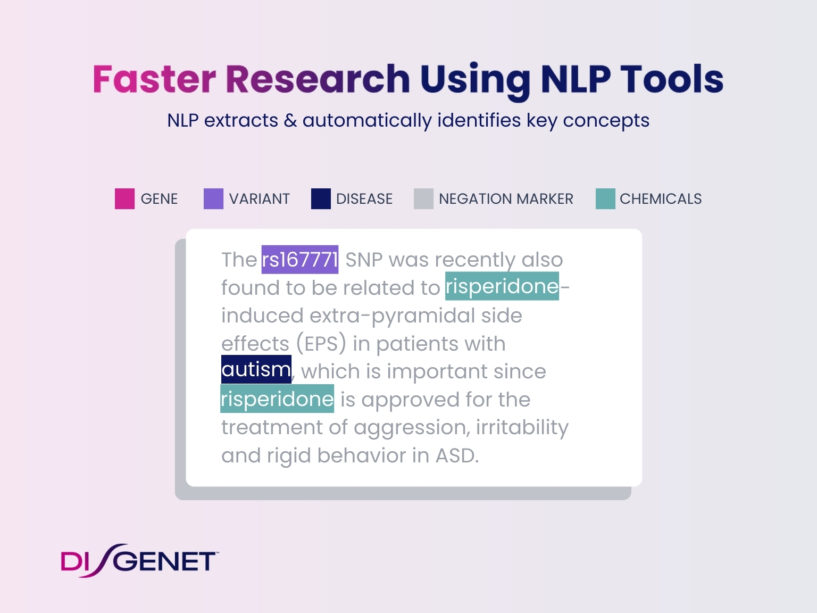
By applying our first and last years of publication filters, you can quickly evaluate years of research, visualize publication trends for a specific association, or directly access the latest discoveries. DISGENET also annotates gain and loss of function mutations associated with disease phenotypes. As a result, you can accurately estimate the potential effects of targeting the related genes.
Additionally, we created two indexes to analyze gene-disease associations. The Disease Specificity Index (DSI) evaluates whether a gene or variant is linked to multiple diseases or only a few. The Disease Pleiotropy Index (DPI) determines whether the associated diseases are similar or span different MeSH disease classes. Thus, target prioritization is enhanced, allowing for more effective and focused therapeutic strategies.
Finally, our high-quality data is the perfect input to train AI/ML tools and fill in the vastly incomplete gene-disease association network to bring to light new therapeutic opportunities in drug discovery [9].
DISGENET, with the power of NLP, will enable you to uncover critical information, significantly speeding up drug development.
Sign up here for a free 7-day trial of DISGENET Advanced
Request a Free Demo here
Contact us at info@disgenet.com
References
[1] Landhuis, E. (2016) Scientific literature: Information overload. Nature, 535, 457–8. https://doi.org/10.1038/nj7612-457a
[2] Siegel, M.G., Rossi, M.J. and Lubowitz, J.H. (2024) Artificial Intelligence and Machine Learning May Resolve Health Care Information Overload. Arthroscopy: The Journal of Arthroscopic & Related Surgery, 40, 1721–3. https://doi.org/10.1016/j.arthro.2024.01.007
[3] Liu, Z., Roberts, R.A., Lal-Nag, M., Chen, X., Huang, R. and Tong, W. (2021) AI-based language models powering drug discovery and development. Drug Discovery Today, 26, 2593–607. https://doi.org/10.1016/j.drudis.2021.06.009
[4] Bhatnagar, R., Sardar, S., Beheshti, M. and Podichetty, J.T. (2022) How can natural language processing help model informed drug development?: a review. JAMIA Open, 5, ooac043. https://doi.org/10.1093/jamiaopen/ooac043
[5] Cook, D., Brown, D., Alexander, R., March, R., Morgan, P., Satterthwaite, G. et al. (2014) Lessons learned from the fate of AstraZeneca’s drug pipeline: a five-dimensional framework. Nature Reviews Drug Discovery, 13, 419–31. https://doi.org/10.1038/nrd4309
[6] Nelson, M.R., Johnson, T., Warren, L., Hughes, A.R., Chissoe, S.L., Xu, C.-F. et al. (2016) The genetics of drug efficacy: opportunities and challenges. Nature Reviews Genetics, 17, 197–206. https://doi.org/10.1038/nrg.2016.12
[7] Minikel, E.V. and Nelson, M.R. (2023) Human genetic evidence enriched for side effects of approved drugs [Internet]. https://doi.org/10.1101/2023.12.12.23299869
[8] Nosengo, N. (2016) Can you teach old drugs new tricks? Nature, 534, 314–6. https://doi.org/10.1038/534314a
[9] Bonner, S., Barrett, I.P., Ye, C., Swiers, R., Engkvist, O., Bender, A. et al. (2022) A review of biomedical datasets relating to drug discovery: a knowledge graph perspective. Briefings in Bioinformatics, 23, bbac404. https://doi.org/10.1093/bib/bbac404